A modification of the bootstrapping soft shrinkage approach for spectral variable selection in the issue of over-fitting, model accuracy and variable selection credibility



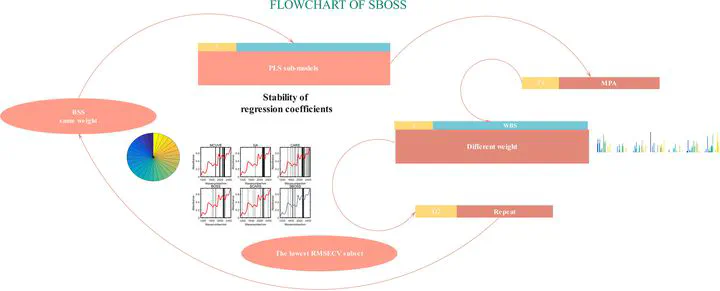
Abstract
In this study, we proposed a new computational method stabilized bootstrapping soft shrinkage approach (SBOSS) for variable selection based on bootstrapping soft shrinkage approach (BOSS) which can enhance the analysis of chemical interest from the massive variables among the overlapped absorption bands. In SBOSS, variable is selected by the index of stability of regression coefficients instead of regression coefficients absolute value. In each loop, a weighted bootstrap sampling (WBS) is applied to generate sub-models, according to the weights update by conducting model population analysis (MPA) on the stability of regression coefficients (RC) of these sub-models. Finally, the subset with the lowest RMSECV is chosen to be the optimal variable set. The performance of the SBOSS was evaluated by one simulated dataset and three NIR datasets. The results show that SBOSS can select the fewer variables and supply the least RMSEP and latent variable number of the PLS model with the best stability comparing with methods of Monte Carlo uninformative variables elimination (MCUVE), genetic algorithm (GA), competitive reweighted sampling (CARS), stability of competitive adaptive reweighted sampling (SCARS) and BOSS.
Publication
Spectrochimica Acta Part A: Molecular and Biomolecular Spectroscopy
Type
Journal Articles